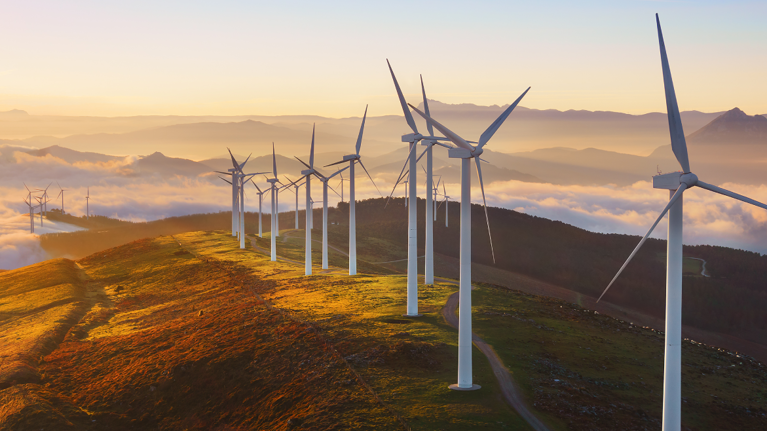
The Power of Data: Can We Meet Data Centers Energy Demand?
Do we see a disruption in energy consumption for data centers and networking – and what does it mean?
Over the last decade, various alarming articles have been published on the energy consumed by data centers and telecommunication networks, painting the picture of drained power plants to sate infrastructure hunger for energy.
This research was based on the estimated exploding amount of data generated and passed across networks, such as the Cisco Visual Networking Index. Simply put, the initial assumption reported by several authors was that if volumes of data grew by 10X, the energy consumed would increase tenfold too. Later, more detailed studies disproved this theory, and projections evolved into more accurate ones. There are several reasons why energy consumption did not match the initial forecast, which we will discuss in this article, together with the current state of things in the industry.
Why is it that energy consumed did not explode with the amount of data?
Reason 1: Energy consumed is non-proportional to “work”
The first reason why the consumed energy did not skyrocket is that energy consumption in servers and network equipment is not proportional to the amount of data being processed.
Modern servers are evolving to have a better proportion of used energy to produced work (as that is a desired feature), but there is still a long way to go. Some servers consume as little as 20% of their maximum energy when idle and being proportional from there. But with “a performance mode” configured, the idle consumption is more likely to sit around 50%. Servers have also become more efficient in the amount of energy being used for a particular unit of work – i.e., improving work/energy ratio.
For networking, the correlation is even less prominent, with for example, optical equipment being basically flat. For wireless networking the proportionality is more like servers. The relationship between “work” and energy consumed in case of networking is per network node (illustrated in the diagram below). It implies that if the network needs to be densified so that the number of nodes increases, the energy consumed for the entire network goes up. In other words, networking on a wide area highlights the ratio that is not exposed by individual nodes under such preconditions.
Reason 2: Data center energy consumption is subject to efficiency improvements
The second factor in disrupting earlier projection is that servers and networking equipment is gradually becoming more efficient. Today, the energy consumed for processing or transferring a certain amount of data is less compared to earlier versions of the products. It typically still exhibits the same idle and work-related un-proportionality but with a lower energy consumption level.
Energy consumption is surging due to hungry AI workloads
So, even though data generated yearly exploded from 15 zettabytes in 2015 to 60 zettabytes in 2020, energy consumption of data centers and networking has stayed basically flat thanks to improved hardware efficiency (data/energy ratio), better DC efficiency (technology & control), and economies of scale as outlined by previous sections.
This significant change allowed data center operators to keep their processing centers in existing locations, where energy availability otherwise would be a challenge. That is often in and around major networking hubs and population centers such as Amsterdam, Paris, or London.
IEA has recorded growing energy consumption by data centers since 2020, and projects this trend to continue. In worst-case scenarios the amount of energy can even double from 460 TWh consumed in 2022 to over 1000 TWh in 2026. The projected rise is due to multiple reasons, with the emerging rise of Artificial Intelligence considered one of the main drivers, whilst still counting in continued efficiency gains as outlined in earlier parts of this blog. Most forms of AI are very compute-hungry, as well as data-hungry. AI workloads often run on very powerful devices, such as GPUs that require a lot of energy.
Data center operators are looking at nuclear to address the energy supply issue
In energy-constrained deployments, data center operators are now looking for new approaches. Recently, Microsoft has issued an initiative to re-open the Three-mile-Island nuclear plant to provide the much-needed electricity. Such a re-opening makes sense in the shorter timeframe. There are similar plans voiced by other hyperscalers that opt for nuclear power to meet their energy demands.
Nuclear option is now considered alongside investments in wind-, hydro-, and solar-based energy. Besides new power sources, tech giants work on making backup and energy storage solutions more efficient that can cater for variability in production.
All these initiatives are to combat the surge in energy demand, while still complying with the hyperscalers’ commitments for net-zero carbon emissions, albeit in the future.
Can we utilize data centers smarter to cut peaks in energy demand?
The need to factor in both data center energy availability and networking
In the same way, as data center operators are expecting a disruption in capacity demand driven by AI workloads, we could expect a disruption in the need for data transport. The cloud trend relies largely on the assumption that there is an “almost free” or unlimited data transport capability available.
Growing AI workloads come with a corresponding increase in data transported, perhaps up to a point where data transfer can no longer be assumed free or effortless. With an increasing amount of the handled data, the risk of congestion and drop in quality needs to be considered. This may in turn require a densification of the data network, with a consequent increase in energy consumption.
Energy-aware workload placement is a key concept
When running into a situation where computing depends on a potential short-of-supply energy, some of which is caused by the computing itself, we could expect an even greater fluctuation in spot energy prices than today. As a result, prices and even availability of computing may vary based on the energy supply. There would be a growing need to dynamically shift workloads to places where energy is more available.
Altogether this situation would create follow-the-sun, follow-the-wind schemes, or just urge cloud providers to move anything that can be moved to places with the availability of hydro- or nuclear-based energy. Such energy-based spot market prices would become a new mechanism to control data center load (or more correctly energy consumption). This new approach would include the more dynamic workload placements that could enable data centers to contribute to the regulation of the grid. Further, it could also contribute to a more sustainable future, should energy prices reflect how CO2-friendly the energy has been produced.
Such a macro-level control mechanism could interoperate with the data center internal optimization for a better placement of workloads .
We could view these scenarios through the lens of edge computing. In edge computing, we are often talking about latency as the upper bound a workload can tolerate. The workloads are pushed towards the edge of the networks, addressing the workload constraints.
For an energy-aware workload placement we would have a similar approach, but translated into “How far away can my workload be?”, “Where is energy available, and can my workload run that far away?”
The sum of this is that we are perhaps facing a situation where workloads need to be placed based on both the energy situation and the load on data transport networks. It will then form the need for a more holistic and optimized control of the computation infrastructure layer.
Conclusion
It seems apparent that the data center industry is facing new challenges with the advent of artificial intelligence. Until now, the industry has been able to combat an exploding amount of data being generated while keeping energy consumption rates reasonable.
But recently, this fragile balance has been broken. This paper outlines the basic mechanisms and argues that a more dynamic and intelligent workload placement is needed to address the issues going forward.
Tietoevry is a leading developer of solutions for the data center industry and telecommunications. Among our clients are network equipment providers, silicon providers, service providers, and enterprises.
Reach out to our experts to help you in addressing the need for a more efficient infrastructure supporting your business.
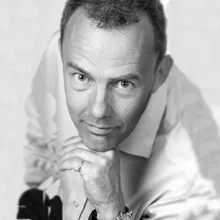
Mats Eriksson leads business development and sales in the telecom and radio access sector in Tietoevry Create. He has previously co-founded technology companies and held managerial positions in various companies. He has a background in academia where he was in charge of a research cooperation institute and founded an EU innovation initiative.